Jul 6: Use of machine learning for early recognition of sepsis at the emergency department
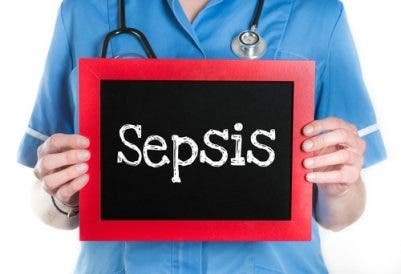
Use of machine learning tools is an interesting and promising approach to develop diagnostic tools for the early recognition of sepsis. In addition, it may also be used for other aspects of data research, such as the identification of diagnostic predictors and the related importance. According to Titus de Hond (UMC Utrecht) in his PhD thesis, this approach may not only be applicable in the context of sepsis, but also for other syndromes that lack a reliable gold standard.
Sepsis is a clinical condition in which an infection causes organ damage. This organ damage is caused by an excessive response of the immune system. Globally, annual sepsis prevalence is estimated at 50 million people and approximately 11 million deaths are sepsis-related annually. Early recognition of sepsis is known to improve outcome rates. Nonetheless, early recognition of sepsis is challenging due to the heterogeneous presentation of sepsis patients. Patients often have non-specific clinical signs and frequently lack signs of a potentially life-threatening disease. In his PhD thesis, Titus de Hond (Department of Acute Internal Medicine, UMC Utrecht) investigated several topics concerning early recognition of sepsis at the emergency department (ED).
Heterogeneity of data
Several issues concerning heterogeneity within sepsis studies were discussed. Unfortunately, it is hard to define sepsis at the emergency department, resulting in varying definitions. Depending on the chosen definition of sepsis, incidence rates differ significantly, which hampers study comparison and generalizability. De hond advises therefore that sepsis research at the ED is likely to benefit from more uniformity between studies.
Biomarkers
Several innovative biomarkers to diagnose sepsis were investigated. Unfortunately, it is likely that the perfect sepsis biomarker doesn’t exist, although some biomarkers show increased clinical value compared to the currently used markers such as c-reactive protein (CRP) and white blood cell count.
Machine learning
The last part of De Hond’s thesis involved research on the value of artificial intelligence and machine learning to diagnose sepsis in an early phase. Using machine learning and data from 375 patients included in the SePsis in the ACutely ill patients in the Emergency department (SPACE) cohort, the investigators identified potential groups of predictors for sepsis diagnosis in routine electronic health record data, including new advanced hematological variables. Routinely collected variables from multiple sources already show predictive power for sepsis diagnosis, indicating that there is already value in data currently collected at the ED. Altogether, this method is an illustrative example of how machine learning can be utilized in data research. According to De Hond, machine learning should not only be used to develop diagnostic tools, but also for other aspects of data research, such as the identification of diagnostic predictors and the related importance. This approach is not only applicable in the context of sepsis, but also for other syndromes that lack a reliable gold standard.
PhD defense
Titus de Hond (1993, Nijmegen) defended his PhD thesis on July 6, 2023 at Utrecht University. The title of his thesis was “Early recognition of sepsis at the emergency department.” Supervisor was prof. dr. Karin Kaasjager (Department of Acute Internal Medicine, UMC Utrecht) and co-supervisor was dr. Jan Jelrik Oosterheert (Department of Infectious Diseases, UMC Utrecht). In October 2022 Titus de Hond started his training to become an internal medicine specialist at Meander MC (Amersfoort).
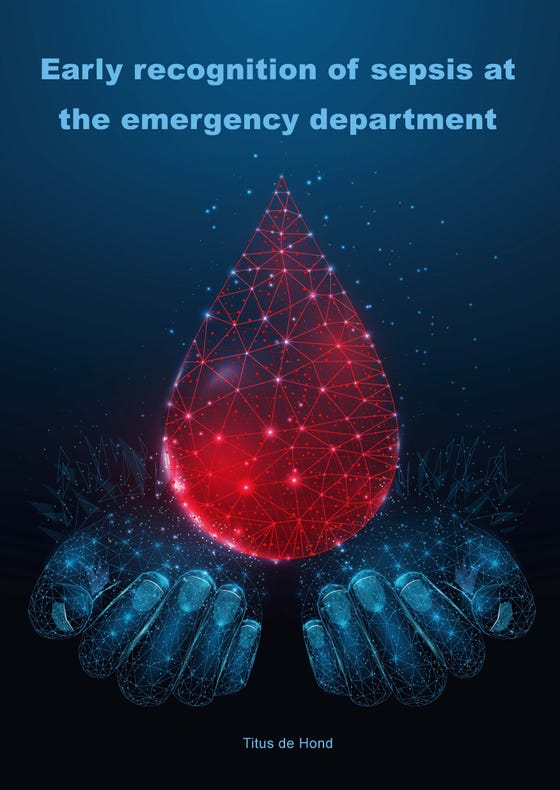